
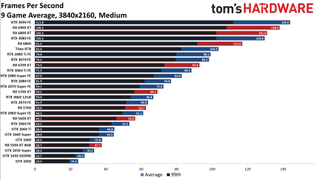

These allow LSTMs to learn highly complex long-term dynamics in the input data and are ideally suited to financial time series learning. They introduce an input gate, a forget gate, an input modulation gate, and a memory unit. Long-Short Term Memory Models (LSTMs) is a specialised form of RNNs designed to bypass this problem. The vanishing gradient problem prevents RNNs from learning longer-term temporal dependencies. Due to the feedback loop, small gradients vanish quickly and large gradients increase dramatically. This occurs during the back-propagation algorithm used for training, where gradients of the cost function are calculated backwards from outputs to inputs. RNNs have difficulties learning long-term dependencies in the inputs due to the vanishing / exploding gradient problem. In this post, we compare the performance of the Nvidia Tesla P100 (Pascal) GPU with the brand-new V100 GPU (Volta) for recurrent neural networks (RNNs) using TensorFlow, for both training and inference. However, since most of the applications involve financial time series as inputs, the recurrent neural network (RNN) model is a central deep learning model for finance. Many different deep learning models have been developed for these applications, including feed-forward neural networks or convolutional networks. Application areas include algorithmic trading, risk management, economic impact studies, asset allocation, and more. The mainstream has primarily focused on applications for computer vision and language processing, but deep learning also shows great potential for a wider range of domains, including quantitative finance. It has shown outstanding performance in solving a wide variety of tasks from almost all fields of science. Artificial intelligence, and in particular deep learning, has become hugely popular in recent years.
